Accelerating Quantum Futures
Author: Andrew Briggs
April 2022
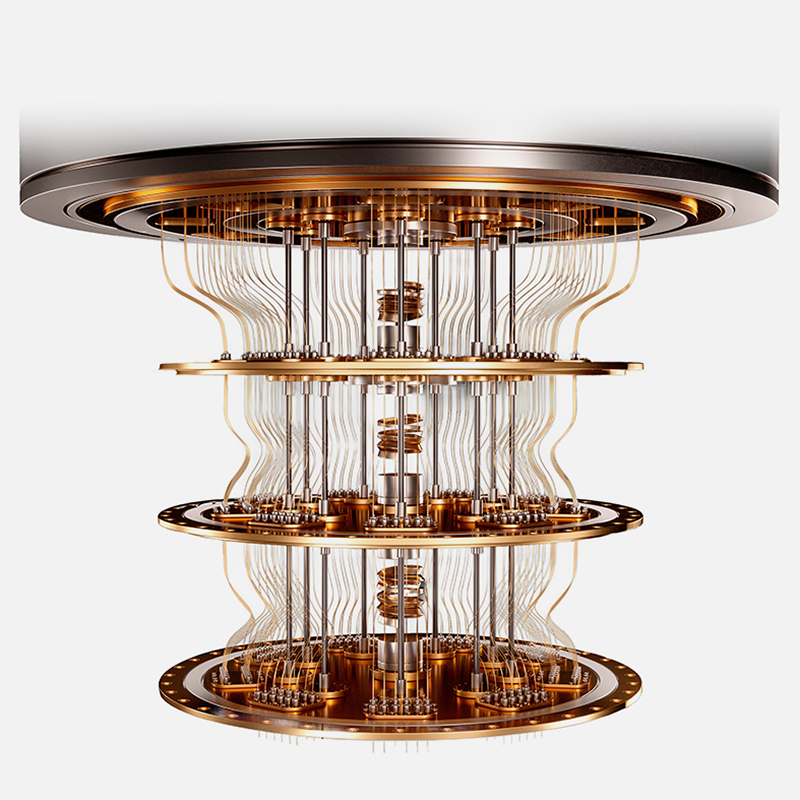
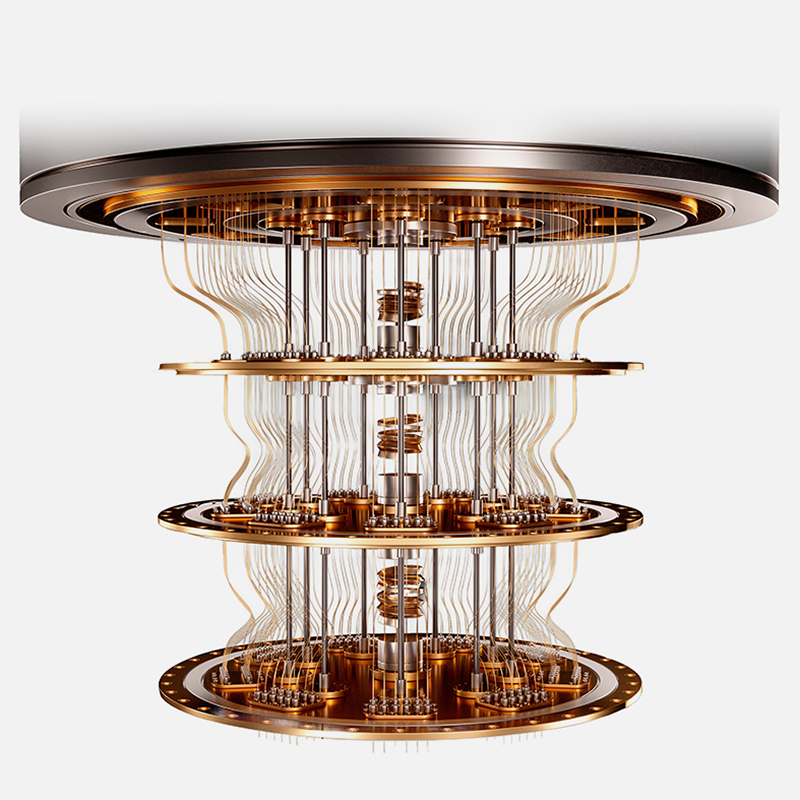
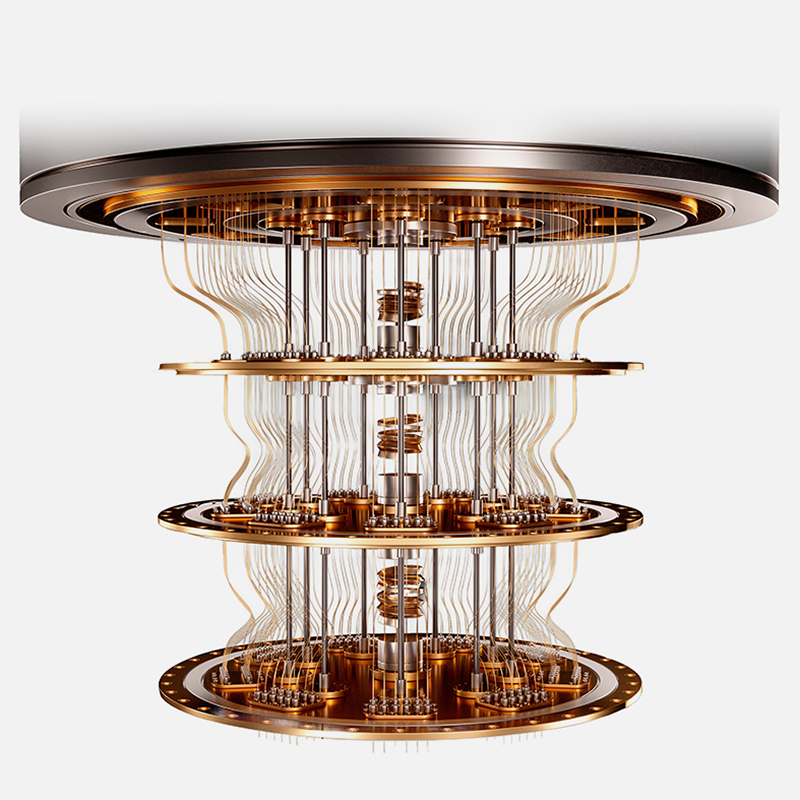
In 2002 EPSRC invited me to take on the directorship of the UK national Quantum Information Processing Interdisciplinary Research Collaboration (QIP IRC), with a mandate to maintain the already strong effort in theory and establish world-leading experimental activity. This was a wise strategy, and QIP IRC contributed significantly to the capacity for quantum technologies in the UK and beyond. I later counted over 40 people who had been appointed to faculty posts in UK universities following participation in QIP IRC. When I took on the role, I asked myself how might QIP IRC fail? It would not be because we could not develop the theory, since that was already well established, including the theory of fault tolerant error correction. But we might fail to get the experiments to work. By the time I stepped down at the end of 2009 there had been such remarkable progress with the experiments—world-wide, not just in the UK—that there was no doubt in my mind that scalable quantum computing would indeed be feasible. The risk was no longer that the experiments might not work, but rather that the engineering of robust quantum computers might prove too difficult. Progress since then has shown that that fear to be unfounded, and we can confidently look forward to successful developments of quantum computing hardware, albeit with uncertainties in the timing and the extent to which there will be a quantum Moore’s Law.
Twenty years ago there were several candidates for implementing qubits. The earliest entry had been ion traps, but there were already good ideas and some demonstrations for spins (nuclear and electron), superconductors (charge, flux, and phase), and photons, with some exciting suggestions such as electrons on helium and NV- centres in diamond. It seemed too early to say which would prove to be the winner, or whether a new idea might come which would trump them all. In some ways it felt like the early days of powered flight when there were hotly contested arguments about whether a biplane or a triplane was better, with the suggestion of a monoplane seeming laughable. It seemed more likely that different aspects of quantum information processing would require different implementations, as we have with classical digital information. That is why QIP IRC focussed on transferring quantum information from one form to another, which was to prove crucial for the subsequent EPSRC hub in Networked Quantum Information Technologies.
Many of the big announcements in scalable quantum computing have used superconducting qubits, mostly variations of the transmon developed some fifteen years ago at Yale University. At least one 1,000 qubit device is scheduled to be completed within the next 2 years. The big announcements have come from USA and China. But there are important European players with some clever ideas which may enable them to outthink the competition. The desire for technological sovereignty has helped to release significant government funding. If there is a technology that is crucial to national security—and before long quantum computing may become such a technology—then you had better satisfy one of three requirements: (i) you can produce it yourself; or (ii) you can purchase it from a wide choice of suppliers; or (iii) you can obtain it from a single source in whom you can have long-term trust. If none of these applies, then you had better go back to (i) and learn how to make it yourself. That may be why in addition to European Innovation Council funding, so many countries are wanting their own flagship quantum computing programme rather like a national airline.
It is far from certain that superconducting quantum computing will be the only game in town. In the USA, the market has put high values on companies which are developing both ionic and photonic quantum machines. Two of the earliest schemes for solid state quantum computing involved spins in semiconductors. These have seen a slow pathway to scalability, but they have various advantages—not least compatibility with established silicon processing—that may one day prove to be winning.
The issue of Nature for 20 January 2022 carried three separate articles demonstrating qubit operations in silicon devices with more than sufficient fidelity for an error-correcting code. This was a landmark for silicon qubits as serious candidates for scalable quantum computing. Rather remarkable was the high degree of cooperation between the groups responsible for the three papers, who might otherwise be considered as competitors. It was a shining example of how, even in such an intense field as quantum computing, humans can gain far more by cooperating than by competing.
Bob Sorensen of Hyperion Research expressed the need forcefully, “There are simply not enough qualified people around to build multiple full-stack quantum computing companies.” Perhaps some like Google and IBM might try to go it alone, but others will struggle. Ultimately, the challenge going forward for the industry to scale will be to create an ecosystem and supply chain in which specialist companies can focus on their core strengths. These ecosystems will lead innovation and drive down costs so that in the next 5-10 years the world will have not 10s of quantum computers but thousands and possibly even 10s of thousands of quantum computers. Such ecosystems are starting to emerge in Europe with Delft leading the way. Some fantastic achievements include QuantWare’s Contralto is a 25 superconducting qubit processor which apparently is available with a 30 day lead time, and Qblox’s control hardware and system integration capabilities of Orange Quantum System. Bluefors provided a dilution fridge for the testbed where companies plug in and test their components. I am told that it took the team just 3 months to bring up the quantum computing testbed from scratch, which would represent a staggering achievement. Organisations from around the world have looked at the Delft model and are seeking to replicate that. I am aware of similar open-test bed projects starting in Finland, India, and other countries. In Europe we don’t have the funding of the IBMs and Googles or the Chinese government. We need more Delft-like ecosystems. BusinessQ in Finland is another ecosystem in early stages of development, where there is strong push by VTT to develop an open test-bed where any company can plug-in their QPUs to test and benchmark their hardware and software.
In the News & Views piece covering the three papers in that issue of Nature, two scientists from Virginia Tech appraised that the achievements of all three groups move silicon-based quantum-information processing a step closer to offering a viable quantum-computing platform. But they then cautioned that a lot of the qubits’ calibration, benchmarking and achieved fidelities will be negatively affected when the system size is increased — even by a single qubit. “At some point, adding qubits to the array will do more harm than good.”, they wrote, “This is because it will become too difficult to calibrate and control a large system with multi-qubit interactions.” This will also be true of other implementations, whether or not they are solid state.
Seven years ago, the Advisory Board for my laboratory, chaired by Dr Hermann Hauser, recommended that we should develop machine learning for our quantum experiments. This proved to be a challenging undertaking, and at first we fumbled around somewhat without really knowing how to make use of all the available techniques. But with a lot of hard work, and high level input from colleagues in Oxford who are pioneers in their fields, we gradually became ever more proficient. A series of brilliant postdocs and graduate students have worked together to produce what we believe is the most advanced software in the world for learning to tune and characterise solid state qubits. The results are astonishing. In one trial, without any reprogramming our software could learn to tune three utterly different architectures for silicon and silicon-germanium single-triplet devices, in each case faster than our benchmark of experienced humans.
Our methods are already providing their value in five different laboratories in Europe and Australia. For early-stage hardware companies, the ability to tune efficiently will be essential for competing with the big players for scalability, fidelity, and maintaining up-time, and the ability to characterise efficiently will be essential for expediting feedback for further development of the design and fabrication of ever more complex circuits. Machine learning is more scalable than human expertise, and offers endless scope for accelerating quantum futures.
What is the challenge going forward? If the threat in 2002 was that the experiments would not work, the threat now is that we might fail to cooperate to make progress together faster than we could separately. There is no doubt in my mind that scalable quantum computers will be built. Investors are hard at work choosing which companies to back. There is growing interest in sectors from fintech to transport to be the first to make use of quantum computing for their optimisation problems. An exciting place to look for breakthroughs is in application specific quantum processors for each family of problems. Hardware development and algorithm development will be the left foot and the right foot on which quantum computing will advance. I look forward to watching, and in a focussed way contributing to, the acceleration of quantum progress.
About the author: Professor Andrew Briggs is the Executive Chair and co-founder of QuantrolOx. He is the inaugural holder of the Chair of Nanomaterials at the University of Oxford. His research interests focus on materials and techniques for quantum technologies and their incorporation into practical devices.